Confabulations and the Art of Machine Learning
We are at a new crossroads in which the machine can either be our servant or master. They have the potential to be disruptive, to change patterns of work, service and play. The test is whether machine learning, like all technology, is used wisely.
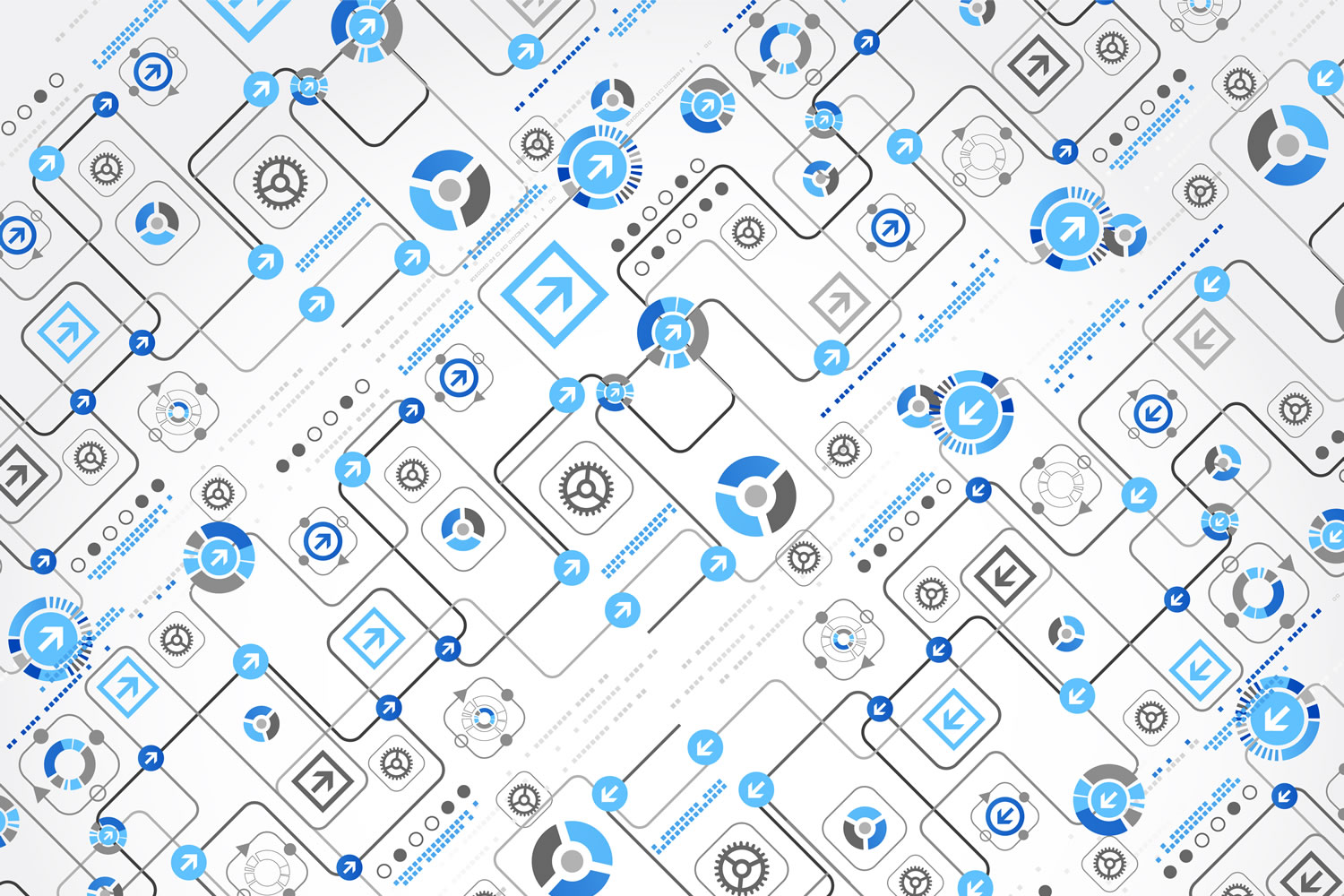
John Berger, Art Critic and Social Commentator
I have always been an admirer of John Berger, the art critic and social commentator most noted for his seminal book Ways of Seeing published in 1972, who has died earlier this Year aged 90. I loved his pictorial collaboration with the photographer John Mohr on the life of European migrant workers in the Seventh Man (1975); and his evocative account of living and farming in the disappearing world of rural France captured in Pig Earth (1979). His work always invites us to see the world differently, and thus encourage us to move in new directions. He says in Ways of Seeing: “What we see and what we know is never settled”*
His final book of reflections published just before he died he entitled ‘Confabulations’. The OED translates this as ‘private and friendly conversations’, while the LOD suggests it means ‘talking together’. This clearly suggests intimacy and warmth, gossip and chatter, something collaborative, conversations about what is personally significant to us, dialogue perhaps of agreement or disagreement: out of which we make sense of our world. Here Berger returns to one of his key themes, that of language and its use.
As consultants we spend a good deal of our time as translators between different worlds.
As a consultant often working to mediate between agencies in complex settings and dealing with people who can feel at great distance from the professionals seeking to support them language and its use has always been the critical tool for me. Tools and techniques maybe useful: but it is the conversations that help us to decide when and how to use these tools, and pull out the messages that arise, that is the meat-and-drink of any good consultant. As consultants we spend a good deal of our time as translators between different worlds.
“True translation”, Berger says in Confabulations, “is not a binary affair between two languages but a triangular affair: the third point of the triangle being what lay behind the words of the original text. True translation demands a return to the pre-verbal” **. Getting behind what a speaker is trying to say, understanding the context and desire, trying to learn their starting points and assumptions is more than words: it is also critical to see gesture and hear voice inflection, to see smile or a shed tear, to ‘feel’ the mood and ambience.
Here I used to think that technology had its limitations, particularly as a ‘return to the pre-verbal’ and therefore the intuitive can often involve group collaboration and discourse in the same physical setting. Yet I see that recent advances in machine learning are starting to help us build on the intuitive. For example a US healthcare organisation called Triggr that supports people in recovery from addictions have devised a new app for mobile phones that uses algorithms *** to map deviation from safe behaviours and so trigger a human intervention before someone stops engaging in positive recovery behaviour. As the machine learns it builds up a greater degree of predictability, and now boasts over 92% accuracy.
I am fascinated by this new machine world, with its ability to manage complex data and pull out messages for us to use in making sense of ‘the pre-verbal’. I can see enormous benefits to improving public service. These developments represent the ongoing, natural tension in the partnership between the human and the technical with which we constantly grapple: but a partnership that must always remain at the service of humans. At Triggr medical experts and ex-addicts define and review what constitutes safe behaviours and risk moments, against individual starting profiles: the machine then ‘manages’ data that contributes to the case management and well-being of an individual by triggering the most appropriate human intervention based on reproducing what the CEO calls ‘motherly intuition’.
Researching the term ‘confabulations’ further I was amazed to learn that it is central to the theory of cognition and consciousness advanced by S.L. Thaler, an American scientist, in the 1990s in which he shows that new thoughts and ideas can originate in artificial neural networks (ANNs) in the same manner as biological (animal/human) networks i.e. there are emergent ideas in machines as well as in humans that can arise from learning.
In psychiatry ‘confabulation’ is defined as a disturbance of memory, in which the ‘speaker’ produces fabricated, distorted, or misinterpreted memories about oneself or the world, but importantly without the conscious intention to deceive. These distortions can vary on a continuum between subtle alterations or bizarre fabrications, and in which the individual is generally very confident about their recollections, despite contradictory evidence. Although individuals can present blatantly false information, confabulation can seem to the speaker to be coherent, internally consistent, and relatively normal. (Is this a definition of Trump-ism?)
If I follow the thread correctly, although confess to not fully understanding the science, while confabulation in psychiatry equates to a false, degraded, or corrupted memory, in an ANN confabulation exploits these ‘false memories’ along with other data inputs through neural feedback loops to form the basis of inventive sensemaking systems (such as Triggr). The idea of such opportunistic confabulation seems to have grown out of two sources. Firstly, experiments with ANNs that simulated brain cell apoptosis i.e. the natural and highly regulated process of cell death in humans^ that confers life-cycle advantage, where it was discovered that novel perceptions and the creation of new ideas can arise from either reversible or irreversible neurobiological damage. Secondly it grew out of vector analysis of data retrieval, aided by the growing power of computers, and is currently used to do such things as detect credit card fraud.
So we are standing at a new crossroads in which the machine can either be our servant or master. I now hear some people speculating as to whether these new developments are the basis for a second industrial revolution. They certainly have the potential to be disruptive, to change patterns of work, service and play. The ultimate test is whether machine learning, like all technology, is used wisely, and to the overall benefit of humans. This is where we must heed the Royal Society’s counsel: “Careful stewardship will be needed to ensure that the productivity dividend from machine learning benefits all in society.”^^
In terms of the public sector world I recall a late GP friend cautioning me on the limitations of generic strategies based solely on numbers (using a very apposite phrase in a machine learning age) where: “the algorithmic bastions remain unbreached”^^^: by which he meant in the health care context that there is a hegemony of evidence-based clinical trails across broad populations to determine clinical practice and commissioning behaviour that take no account of what he calls the “personal significance” to a patient of their presenting health circumstances which the doctor helps to interpret. In other words the numbers, the stories and the relationships become disconnected. I am therefore heartened by a machine tool such as Triggr that promotes personal significance, in which the individual interprets data with a recovery expert that, at the same time, builds up a body of general research.
For Berger confabulations enabled him to make sense of his world, to translate between different peoples and contexts, to access the pre-verbal. Machine learning it seems can also use the process of confabulation in the service of humans to access the pre-verbal. We are only at the beginning of this understanding. It seems we all need to confabulate a bit more!
______________________________________________________________________________________________________________________________
* -John Berger (1972), “Ways of Seeing”
** -John Berger (2016), “Confabulations”. Penguin
*** -For the uninitiated, algorithms are defined as a process or set of rules to be followed in calculations or other problem-solving operations, especially by a computer. The word has its roots in an interesting mix Latin (algorismus), Greek (arithmos), Medieval English (algorism) noting the decimal notation of numbers, and Arabic (from al-.warizmi, ‘the man of .warizm’ (now Khiva), a name given to the 9th-century mathematician.
Abu Ja‘far Muhammad ibn Musa, author of widely translated works on algebra and arithmetic.
^ -Between 50 and 70 billion cells die each day due to apoptosis in the average human adult
^^ -Royal Society (2017). “Machine learning: the power and promise of computers that learn by example”
^^^ -Kieran Sweeney (2006). “Complexity in Primary Care: Understanding its Value”. Radcliffe Press